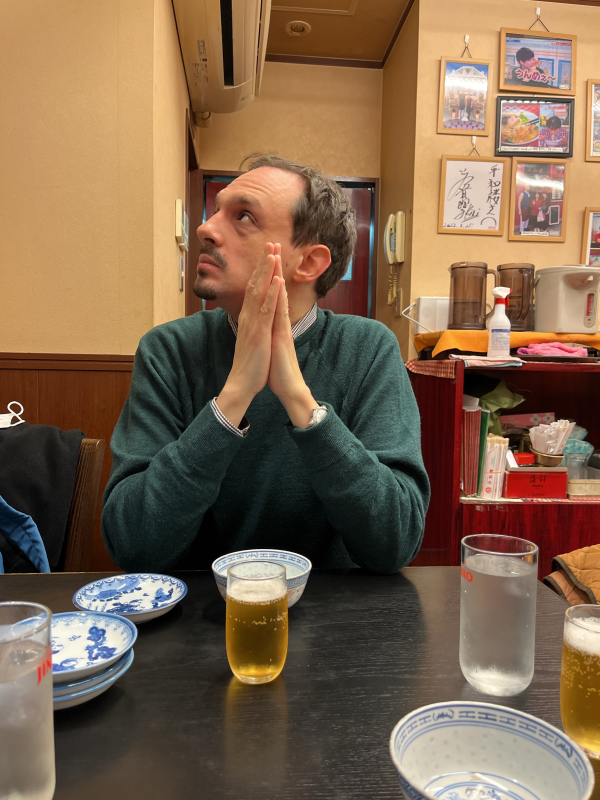
Marco Scutari, Ph.D.
Senior Researcher in Bayesian Networks and Graphical Models
Contact Information
Istituto Dalle Molle di Studi sull'Intelligenza Artificiale (IDSIA)
Polo Universitario Lugano
Via la Santa 1
6962 Lugano (Switzerland)
email: scutari@bnlearn.com
Here are my curriculum vitae, my Google Scholar profile and my arXiv preprints.
Publications
Books
- The Pragmatic Programmer for Machine Learning: Engineering Analytics and Data Science Solutions.
[ CRC |
Amazon |
code, info and errata |
doi ]
M. Scutari and M. Malvestio (2023).
Chapman & Hall.
- Bayesian Networks with Examples in R.
[ CRC |
Amazon |
data, code and errata |
doi ]
M. Scutari and J.-B. Denis (2021).
Chapman & Hall, 2nd edition.
Japanese translation
- Bayesian Networks in R with Applications in Systems Biology.
[ Springer |
Amazon |
data, code and errata |
doi ]
R. Nagarajan, M. Scutari and S. Lèbre (2013).
Use R!, Springer (US).
Older books and book editions are available here.
Book Chapters
Older book chapters are available here.
Refereed Journal Articles
In the works
- fairml: A Statistician's Take on Fair Machine Learning Modelling.
[ arXiv (preprint) ]
M. Scutari.
Journal of Statistical Software. - Abnormal Child Behavior in Primary School Students: a Bayesian Network Analysis.
[ ]
A. C. Till, T. R. Henry, M. Scutari and G. Briganti.
Psychological Reports. - Federated Causal Discovery with Missing Data in a Multicentric Study on Endometrial Cancer.
[ ]
A. Zanga, A. Bernasconi, P. J. F. Lucas, H. Pijnenborg, C. Reijnen, M. Scutari and A. C. Constantinou.
Journal of Biomedical Informatics. - Benchmarking Constraint-Based Bayesian Structure Learning Algorithms: Role of Network Topology.
[ arXiv ]
R. Nagarajan and M. Scutari. TBD. - Technical Insights and Legal Considerations for Advancing Federated Learning in
Bioinformatics.
[ arXiv (preprint) ]
D. Malpetti, M. Scutari, F. Gualdi, J. van Setten, S. van der Laan, S. Haitjema, A. M. Lee, I. Hering and F. Mangili.
Frontiers in Digital Health. - Causal Assessment of Gene Regulatory Networks in Single-Cell Transcriptomics Data Based on Bayesian
Network Using scstruc.
[ ]
N. Sato, M. Scutari and S. Imoto.
Cell Reports Methods.
In print
- Introducing Bayesian Analysis for Clinicians: Gender-Associated Risk Assessment of Intracranial
Aneurysms.
[ doi ]
P. Bijlenga, G. Spinner, M. Scutari, M. Delucchi and S. Hirsch.
Proceedings of the 10th European-Japanese Cerebrovascular Congress. - A Causal Network Model to Estimate the Cardiotoxic Effect of Oncological Treatments in Young Breast
Cancer Survivors.
[ html |
pdf |
doi ]
A. Bernasconi, A. Zanga, P. J. F. Lucas, M. Scutari, A. Trama and F. Stella.
Progress in Artificial Intelligence. This is an extended version of the “Towards a Transportable Causal Network Model Based on Observational Healthcare Data” AIxIA paper. - Information Theory, Machine Learning, and Bayesian Networks in the Analysis of Dichotomous and
Likert Responses for Questionnaire Psychometric Validation.
[ PsyArXiv |
doi ]
M. Orsoni, M. Benassi and M. Scutari.
Psychological Methods.
Published
- Causal Networks of Infodemiological Data: Modelling Dermatitis.
[ arXiv (preprint) |
html |
pdf |
doi ]
M. Scutari, S. Salah, D. Kerob and J. Krutmann (2025).
Proceedings of the 23rd International Conference on Artificial Intelligence in Medicine, Lecture Notes in Artificial Intelligence, 397–407. - Unveiling Sleep Dysregulation in Chronic Fatigue Syndrome with and without Fibromyalgia Through
Bayesian Networks.
[ medRxiv (preprint) |
arXiv (preprint) |
html |
pdf |
doi ]
M. Bechny, M. Scutari, J. van der Meer, F. Faraci, S. Meystre, B. H. Natelson and A. Kishi (2025).
Proceedings of the 23rd International Conference on Artificial Intelligence in Medicine, Lecture Notes in Artificial Intelligence, 33–43 - Causal Modeling in Large-Scale Data to Improve Identification of Adults at Risk for Combined and
Common Variable Immunodeficiencies.
[ medRxiv (preprint) |
html |
pdf |
doi |
online supplementary material ]
G. Papanastasiou, M. Scutari, R. Tachdjian, V. Hernandex-Trujillo, J. Raasch, K. Billmeyer, N. Vasilyev and V. Ivanov (2025).
npj Digital Medicine, 8, 361. - Network Analysis: An Overview for Mental Health Research.
[ html |
pdf |
doi ]
G. Briganti, M. Scutari, S. Epskamp, D. Borsboom, R. Hoekstra, H. F. Golino, A. P. Christensen, O. V. Ebrahimi, A. Heeren, J. de Ron, L. Bringmann, K. Huth, A. Isvoranu, M. Marsman, T. Blanken, T. R. Henry, E. Fried and R. J. McNally (2024).
International Journal of Methods in Psychiatric Research, 33(4), e2034. - From Real-World Data to Causally Interpretable Models: A Bayesian Network to Predict Cardiovascular
Diseases in Adolescents and Young Adults with Breast Cancer.
[ SSRN (preprint) |
html |
pdf |
doi ]
A. Bernasconi, A. Zanga, P. J. F. Lucas, M. Scutari, S. Di Cosimo, M. C. De Santis, E. La Rocca, P. Baili, I. Cavallo, P. Verderio, C. M. Ciniselli, S. Pizzamiglio, A. Blanda, P. Perego, P. Vallerio, F. Stella, A. Trama and the Ada Working Group (2024).
Cancers, 16(21), 3643. - Using Bayesian Networks to Investigate Psychological Constructs: The Case of Empathy.
[ html |
pdf |
online supplementary material |
doi ]
G. Briganti, J. Decety, M. Scutari, R. J. McNally and P. Linkowski (2024).
Psychological Reports, 127(5), 2334–2346. - Inferring Skin-Brain-Skin Connections from Infodemiology Data using Dynamic Bayesian Networks.
[ arXiv (preprint) |
medRxiv (preprint) |
html |
pdf |
doi |
online supplementary material ]
M. Scutari, D. Kerob and S. Salah (2024).
Scientific Reports, 14, 10266. - Learning Bayesian Networks with Heterogeneous Agronomic Datasets via Mixed-Effect Models and
Hierarchical Clustering.
[ arXiv (preprint) |
html |
pdf |
doi ]
L. Valleggi, M. Scutari and F. M. Stefanini (2024).
Engineering Applications of Artificial Intelligence, 31, 107867. - Entropies and Divergences for Bayesian Networks: Computational Complexity and Efficient
Implementation.
[ arXiv (preprint) |
html |
pdf |
doi ]
M. Scutari (2024).
Algorithms, 17(1), 24. - Towards a Transportable Causal Network Model Based on Observational Healthcare Data.
[ arXiv (preprint) |
pdf ]
A. Bernasconi, A. Zanga, P. J. F. Lucas, M. Scutari and F. Stella (2023).
Proceedings of the 2nd Workshop on Artificial Intelligence for Healthcare, 22nd International Conference of the Italian Association for Artificial Intelligence (AIxIA 2023), 67–82. - Machine Learning Software and Pipelines.
[ html |
doi ]
M. Scutari and M. Malvestio (2023).
Wiley StatsRef: Statistics Reference Online. - Developing and Running Machine Learning Software: Machine Learning Operations (MLOps).
[ html |
doi ]
M. Scutari and M. Malvestio (2023).
Wiley StatsRef: Statistics Reference Online. - Analyzing Complex Systems with Cascades using Continuous Time Bayesian Networks.
[ arXiv (preprint) |
html |
pdf |
doi ]
A. Bregoli, K. Rathsman, M. Scutari, F. Stella and S. W. Mogensen (2023).
Proceedings of the 30th International Symposium on Temporal Representation and Reasoning, Leibniz International Proceedings in Informatics (LIPIcs), 8:1–8:21. - A Tutorial on Bayesian Networks for Psychopathology Researchers.
[ PsyArXiv (preprint) |
html |
doi ]
G. Briganti, M. Scutari and R. J. McNally (2023).
Psychological Methods, 28(4), 947–961. - Causal Discovery with Missing Data in a Multicentric Clinical Study.
[ arXiv (preprint) |
html |
doi ]
A. Zanga, A. Bernasconi, P. J. F. Lucas, H. Pijnenborg, C. Rejinen, M. Scutari and F. Stella (2023).
Proceedings of the 21st International Conference on Artificial Intelligence in Medicine, Lecture Notes in Artificial Intelligence, 40–44. - Path Analysis Models Integrating Psychological, Neuro-Physiological and Clinical Variables in
Individuals with Tension-Type Headache.
[ html |
doi ]
B. X. W. Liew, M. Palacios-Ceña, M. Scutari, S. Fuensalida-Novo, A. Guerrero-Peral, C. Ordás-Bandera, J. A. Pareja and C. Fernández-de-las-Peñas (2023).
The Journal of Pain, 24(3), 426–436. - Data-Driven Network Analysis Identified Subgroup-Specific Low Back Pain Pathways: A Cross-Sectional
GLA:D Back Study.
[ html |
pdf |
doi ]
B. X. W. Liew, J. Hartvigsen, M. Scutari and A. Kongsted (2023).
Journal of Clinical Epidemiology, 153, 66–77.
Older papers from 2019 to 2022 are available here.
Older papers from 2015 to 2018 are available here.
Older papers from up to 2014 are available here.
Ph.D. Dissertation and Technical Reports
The material from my Ph.D. dissertation is available here.
Teaching Material
My teaching material is available here.
Invited Talks, (Short) Course Slides, Conference Presentations and Posters
- Bayesian and Causal Networks for Clinical and Epidemiological Data: Concepts, Implementation and
Interpretation.
[ ]
Bayesian Biostatistics Conference (BayesPharma), Leiden (October 22, 2025).
- Causal Networks of Infodemiological Data: Modelling Dermatitis.
[ pdf ]
23rd International Conference on Artificial Intelligence in Medicine (AIME), Pavia (June 25, 2025). - Unveiling Sleep Dysregulation in Chronic Fatigue Syndrome with and without Fibromyalgia Through
Bayesian Networks.
[ pdf ]
23rd International Conference on Artificial Intelligence in Medicine (AIME), Pavia (June 25, 2025). - Causal Modelling for Environmental Epidemiology.
[ pdf ]
Department of Mathematical Sciences, University of Bath (February 11, 2025). Then Department of Computer Science, Brunel University London (February 12, 2025). - Causal Modelling in Space and Time.
[ pdf ]
Department of Systems Innovation, Graduate School of Engineering Science, Osaka University; and online for the Behaviormetric Society (June 18, 2024). And again at the Institute of Medical Science, The University of Tokyo (July 8, 2024). And again at the Data Science and AI Innovation Research Promotion Center, Faculty of Data Science, Shiga University (July 23, 2024). And again at the Brazilian Symposium on Probability and Statistics, Fortaleza (August 5, 2024). And again at a Mathematics webinar organised by MDPI/Sciforum (October 10, 2024). - Distilling Causal Models: Model Averaging, Federated Learning and More.
[ pdf ]
AI and Machine Learning Summer School, Cambridge Centre for AI in Medicine (CCAIM), online (September 2–6, 2024). - bnlearn, Learning Bayesian Networks 15 Years Later.
[ pdf ]
Bayesian Networks Modelling Association (BNMA), online (June 24, 2024). - Fairness in Machine Learning.
[ pdf ]
“Data Science for the Sciences” Conference, Bern (April 11–12, 2024). - Different Takes on the Causal Modelling of Spatio-Temporal Data.
[ pdf ]
Department of Informatics, Systems and Communication, University of Milano Bicocca (March 6, 2024). And again at the Florence Center for Data Science and the Department of Statistics, Informatics and Applications, University of Florence (May 24, 2024). - Network Structures for Psychological Constructs: The Case of Empathy.
[ pdf ]
University Center for Statistics in the Biomedical Sciences (CUSSB), Vita-Salute San Raffaele University, Milan (January 29, 2024). - The Anatomy of a Machine Learning Pipeline.
[ pdf |
online material ]
European Network for Business and Industrial Statistics (ENBIS), online (January 10, 2024). And again at the University of Milano Bicocca (September 16, 2024). - Fair Machine Learning: Achieving Fairness with a Simple Ridge Penalty.
[ pdf ]
Department of Statistics, London School of Economics (June 12, 2023). And again at the Department of Informatics, Systems and Communication, University of Milano Bicocca (June 29, 2023). - Analysing Google Search Trends Data with Dynamic Bayesian Networks.
[ pdf ]
Institute for Global Environmental Strategies (IGES), Tokyo (January 20, 2023). And again at the Department of Systems Innovation, Graduate School of Engineering Science, Osaka University (January 30, 2023). And again at the Bernoulli Workshop on Sparse Inference on Complex Networks, Università della Svizzera Italiana (June 27, 2023). - Bayesian Network Models for Continuous-Time and Structured Data.
[ pdf ]
National Institute of Advanced Industrial Science and Technology (AIST), Tokyo (January 19, 2023).
Older talks from 2019 to 2022 are available here.
Older talks from 2015 to 2018 are available here.
Older talks from up to 2014 are available here.